Named entity recognition and relation extraction for enabling and accelerating drug discovery
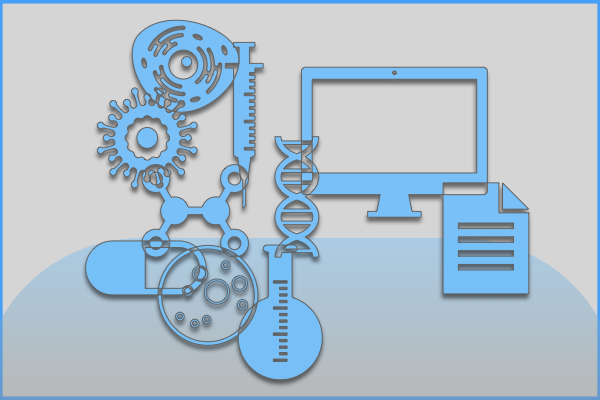
Importance of Identifying named entities and extracting relations
The process of drug discovery is sophisticated and time-consuming. The efficacy of the end-product often comes with the cost of unexpected side effects or toxicity. As a result, the attention of biomedical research changes from individual genes or proteins to entire biological systems. This approach creates a demand for extracting links between bio-molecular entities from published research articles. The discovered knowledge powers the generation of scientific hypotheses for evaluation.
There has been considerable interest in identifying relations between existing chemicals and disease phenotypes for rapid hypotheses generation. Such inventions are vital for improving chemical safety and informing potential relationships between drug molecules and pathologies.
Various tools have enabled text-mining, entity identification, and relation extraction from literature. The methodology used by these tools ranges from co-occurrence-based approaches to complicated machine learning-based strategies. There is a rising interest in developing generalized tools for automatic entity-identification and relation extraction from high-standard, rapidly-growing biomedical literature.
Named Entity Recognition (NER) and Relation Extraction
NER, a natural language processing sub-task, identifies named entities in raw text and classifies them into predefined semantic categories. The categories are defined based on the domain in question. Subsequently, the entities are often normalized and mapped to ontologies or controlled vocabularies. A complementary subtask to NER is relation extraction that targets identifying semantic relationships between the named information highlighted under NER. For example, gene-disease relationships, protein-protein interactions, drug-drug interactions, and clinical problem-treatment relationships biomedicine.Importance of Named Entity Recognition and Relation Extraction
Named Entity Recognition (NER) and Relation Extraction (RE) from medical texts are crucial components of basic and applied scientific discoveries that may influence the drug discovery process. Some of them are summarized as follows:
- Understand disease whereabouts, including demographics, heterogeneity, prevalence, etc.
- Initial target identification and prioritization
- Exploration of the chemical space used for the current target
- Identification of vulnerable cohorts based on genetics, epigenetics, and metagenomics
- Accelerating decision support techniques for the modern healthcare system
Conclusion
Although the potential of using published articles to enable drug discovery is widely known, only recently, only a few pharmaceutical companies are using the full power of pre-existing, published research articles. At Nucleati, we have developed Nucleati Abstracts that provides REST-APIs to access abstracts published in scientific papers. We are the first company to enable end-user to access abstracts published in a given journal in just one API call. Similarly, abstracts published in a given year are just one API call away. The only limitation is the speed of the internet users have. We are also developing powerful tools to extract entities and relations between them and make them available through REST-APIs. All the tools we create are customizable for the various domain of interest and corpora.
Useful external references
1. Improving chemical disease relation extraction with rich features and weakly labeled data. [Article]
2. A two-stage deep learning approach for extracting entities and relationships from medical texts [Article]
3. Text Mining for Drug Discovery [Article]
4. Relation extraction methods for biomedical literature [Article]